Lessons from the transistor
Going back further than mobile, cloud, or the internet might just reveal the best comparison for AI's future
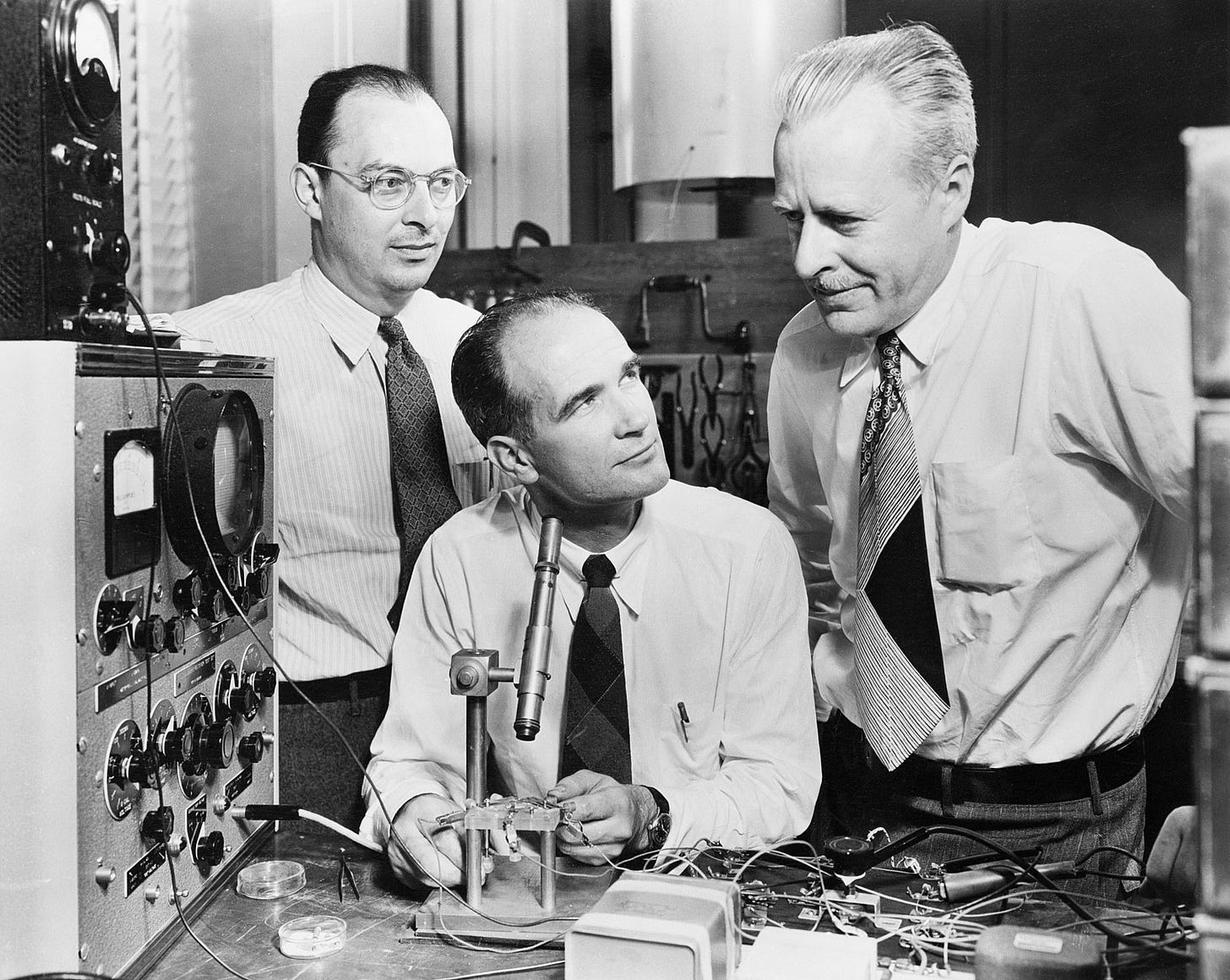
These days, everyone is trying to assess the impact of AI. Recently, I was listening to a podcast that suggested that the transistor may be a fitting analogy.
I knew a bit about the transistor at the time but certainly not enough to make a case either way. So, naturally, I decided to do some homework1.
As it turns out, the story of the transistor is quite insightful. It’s an imperfect analogy (they all are) but there are lessons to be learned.
A little history
What’s a transistor?
A transistor is a device made of silicon that can control the flow of electricity like a switch and amplify electronic signals. It is a fundamental building block of modern electronics, from simple radios to the most powerful computers.
Chris Miller, author of Chip War, puts this eloquently:
“At the core of computing is the need for many millions of 1s and 0s. The entire digital universe consists of these two numbers. Every button on your iPhone, every email, photograph, and YouTube video—all of these are coded, ultimately, in vast strings of 1s and 0s. But these numbers don’t actually exist. They’re expressions of electrical currents, which are either on (1) or off (0). A chip is a grid of millions or billions of transistors, tiny electrical switches that flip on and off to process these digits, to remember them, and to convert real world sensations like images, sound, and radio waves into millions and millions of 1s and 0s.”
Bell Labs: The birth of the transistor
The transistor was invented in 1947 by John Bardeen, Walter Brattain, and William Shockley at Bell Labs. It replaced vacuum tubes, the state of the art at the time, and enabled smaller, more efficient, and more reliable electronic devices.
Ed Eckert, Nokia Bell Labs’ corporate archivist, explains...
“It evolved a primitive device to one that can be made super small…The vacuum tube had its limitations: its size, it used lots of power, it was fragile, it created lots of heat. So, the transistor was the answer to all these problems.”
To illustrate, the left side of the image below shows a single vacuum tube, whereas the far right (#15) shows an integrated circuit with 4,000 to 6,000 transistors in it.
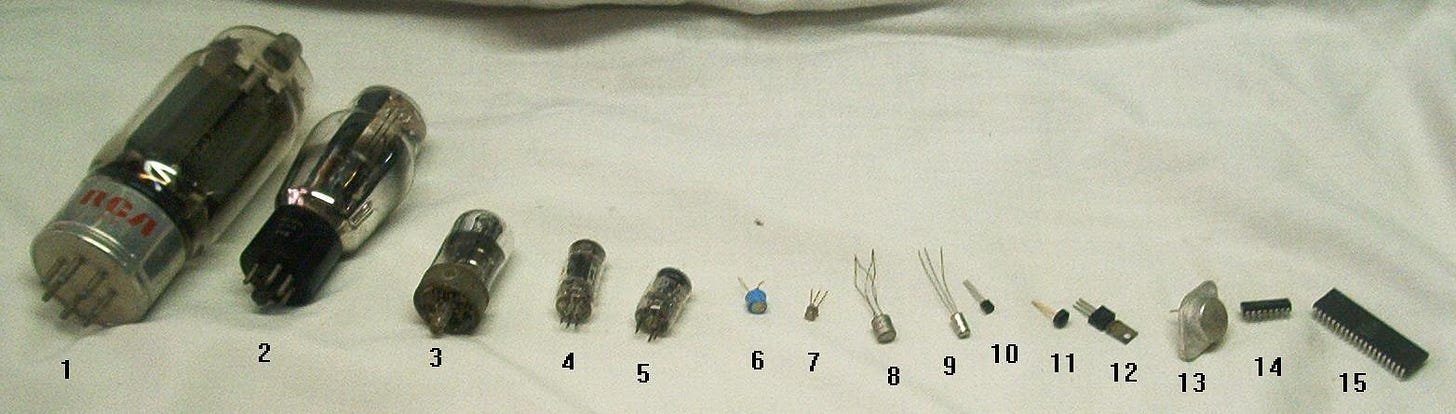
Today, the smallest transistors are measured in single-digit nanometers. For scale, a strand of DNA is 2.5 nanometers and a human hair is ninety thousand nanometers thick. Personal computers, iPhones, or AirPods couldn’t exist with vacuum tubes.
The shrinking of the transistor is, of course, Moore’s law. Moore’s law states that the number of transistors per processor will double every two years…
…which dramatically drives cost per transistor downwards.
The net is (1) much more powerful electronics at any given size (2) cheaper electronics and (3) smaller electronics.
So what?
The transistor was the Big Bang moment for modern electronics. Bill Gates even said:
“My first stop on any time-travel expedition would be Bell Labs in December 1947.”
Every device we rely on today from our phones to our cars to medical devices is now powered by transistors. As a result, the transistor propelled national productivity forward. The St. Louis Fed said in a 2007 report:
Numerous studies have traced the cause of the productivity acceleration to technological innovations in the production of semiconductors that sharply reduced the prices of such components and of the products that contain them (as well as expanding the capabilities of such products).
The transistor has been a pervasive driver of economic growth for decades and likely will continue to be for decades more.
Is the transformer the next transistor?
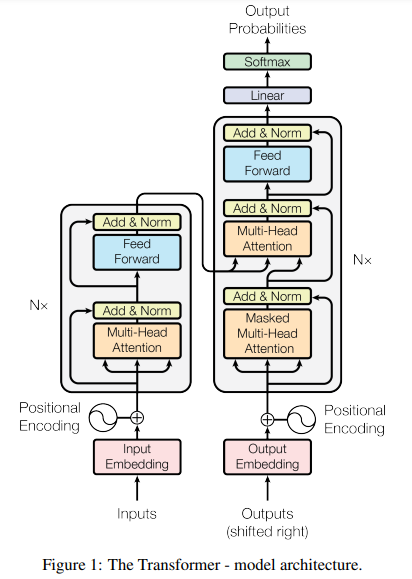
The current AI2 boom traces back to the 2017 paper “Attention is All You Need” which introduced the Transformer Architecture. This is comparable to the 1947 invention of the transistor for the AI timeline.
The history of the transformer is still being written, but there’s already a few similarities between it and the transistor.
The founding story rhymes
Both the transistor and transformer were invented in corporate research labs: the transistor at Bell Labs and the transformer at Google.
Unfortunately for Bell, neither AT&T3 (the former parent) nor Nokia (the current parent) reaped the majority of the rewards of the computing revolution. It’s early, but Google may be headed in that direction with OpenAI and others generating most of the direct4 enterprise value from the transformer.
While the story has been written for Bell, we will see what happens to Google.
Both have had early reliability challenges
LLMs make things up, which unfortunately makes them unreliable for many use cases today. The early transistor was also seen as unreliable.
These issues were eventually solved for the transistor, but it required considerable wrangling from a few visionaries, like Morris Chang:
“A master bridge player, [Morris] Chang approached manufacturing as methodically as he played his favorite card game. Upon arriving at TI, he began systematically tweaking the temperature and pressure at which different chemicals were combined, to determine which combinations worked best, applying his intuition to the data in a way that amazed and intimidated his colleagues. “You had to be careful when you worked with him,” remembered one colleague. “He sat there and puffed on his pipe and looked at you through the smoke.”…Chang’s methods produced results, though. Within months, the yield on his production line of transistors jumped to 25 percent.”
If you’re like me, these systematic tweaks and combinations feel like prompt engineering today. Small and seemingly unscientific adjustments can yield real results.
Check out this OpenAI guide on prompt engineering, for example. Reading tactics like telling the model to use its own inner monologue (see below) is what it must have felt like to watch Morris Chang tweak the production process in ways you could never have imagined.
The big one: scaling laws
The magic of the transistor can’t be decoupled from Moore’s law.
LLMs, based on the transformer architecture, also have scaling laws. This chart is already outdated but illustrates LLM scaling laws since 2018:
This chart implies scaling laws more powerful than Moore’s law - at least over this four year period. Of course, the magic of Moore’s law is its persistence. The trajectory of LLM scaling laws is promising, but time will tell if they can persist as long as Moore’s Law.
Like the transistor, we’re also seeing exponential declines in price alongside improvements in capabilities. This dramatically increases access and adoption of this technology.
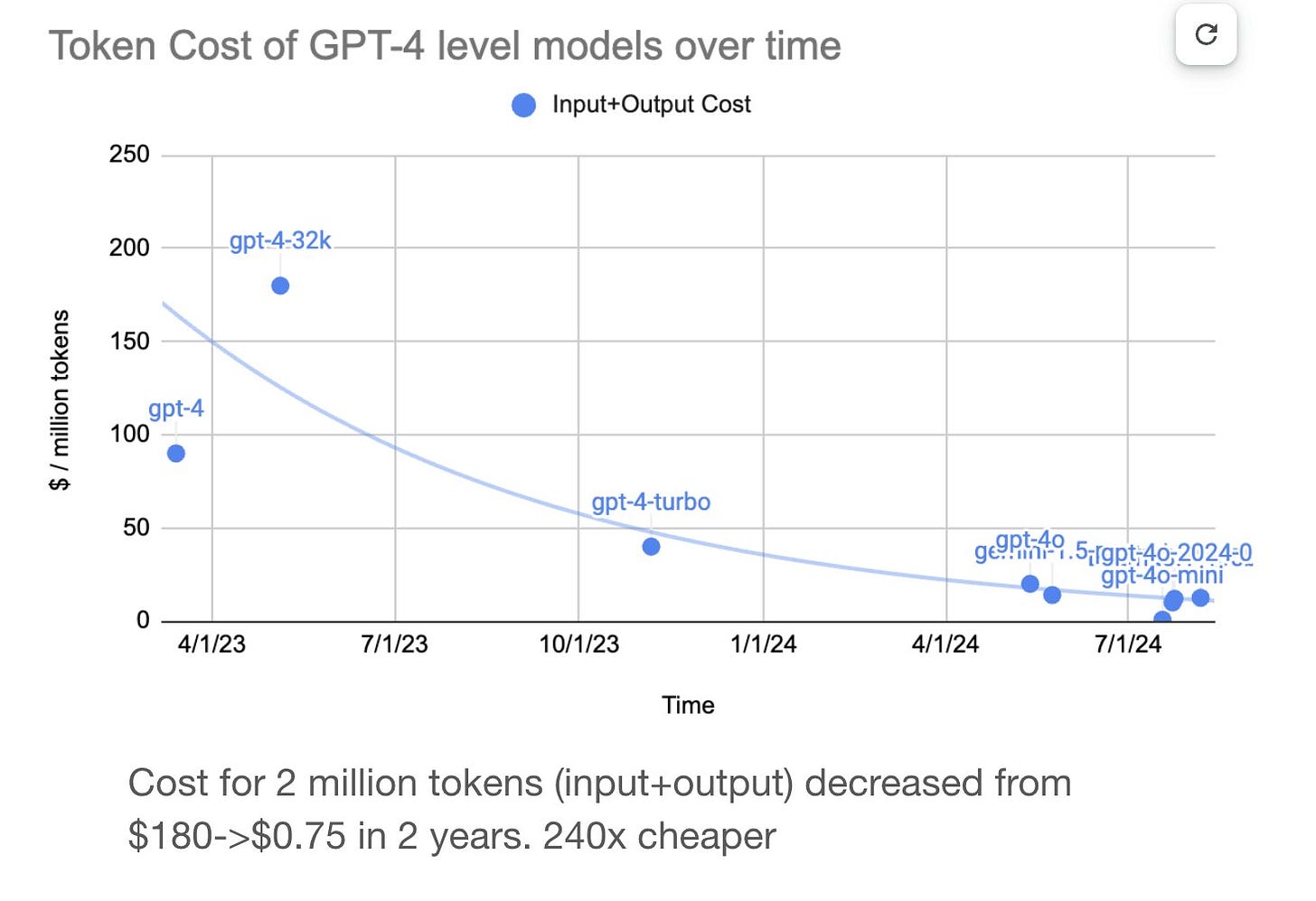
The impact of the transistor can’t be decoupled from Moore’s Law so this is the most important similarity between the transistor and the transformer.
Potential lessons for AI
If the transistor and the transformer share some similarities, what might that tell us about the future of transformer-based AI models?
We can’t comprehend what’s possible
Humans struggle to grasp exponential growth. It’s one thing to look at a line on a graph go up and up and up. It’s another to understand what it represents.
When the transistor was invented, few could foresee what it truly meant for the future of computing.
In 1965, Gordon Moore was one of those people when he boldly proclaimed:
“Integrated circuits will lead to such wonders as home computers – or at least terminals connected to a central computer – automatic controls for automobiles, and personal portable communications equipment”
This was decades before the personal computer and the iPhone.
To put these advances into perspective, the processing power of an iPhone is 100,000 times greater than that of the Apollo 11 computer in 1969.5 The average person in 1969 couldn’t possibly grasp that the processing power of the Apollo 11 rocket would fit in their pocket a few decades later.
Current AI models may be unreliable but they’re improving at a mind-boggling rate, like the early days of the transistor.
Further, AI applications today aim to augment or replace human capabilities, which has the potential to accelerate human productivity (like the transistor). However, the biggest impact of AI will be superhuman capabilities that we can’t even imagine today.
I’m avoiding the three-letter word that starts with A and ends with I on purpose. In 2017, we already saw the power of AI to create new knowledge with AlphaGo’s unprecedented strategic moves. This was without anything close to AGI.
I’m excited to see the novel discoveries we will make using AI in science, medicine, and engineering.
The impact will be ubiquitous
The transistor transformed every sector of the economy.
Take the auto industry: today, the average car has 300-1,000 chips in it. The efficiencies, amenities, and safety features of modern vehicles all originate from the transistor.
The same goes for satellites, medical equipment, TVs, industrial systems, and more. The modern toaster even has microchips for consistent browning. Electronics are everywhere.
AI will have the same level of impact. AI will be embedded everywhere, from vehicles to hospitals to fast food restaurants. You won’t interact with a device or business without interacting with AI in some form.
An early lead can create long term dominance
Over time, the exponential improvements in electronics benefited companies that embraced Moore’s Law early - long before today’s capabilities.
Look no further than the graphics from the Madden NFL video game franchise in 1988 vs 2023.
![MADDEN NFL evolution [1988 - 2023] MADDEN NFL evolution [1988 - 2023]](https://substackcdn.com/image/fetch/w_1456,c_limit,f_auto,q_auto:good,fl_progressive:steep/https%3A%2F%2Fsubstack-post-media.s3.amazonaws.com%2Fpublic%2Fimages%2Ff23ae8cf-2819-484f-9e92-54dd3cb95ae9_1280x720.jpeg)
To today’s gamer, the 1988 version is unplayable. Yet, EA published it anyways because, at the time, it was cutting edge.
As better graphics became possible due to improvements in GPUs, EA has maintained their position as the leading football video game franchise. The takeaway for AI-enabled products is that it’s always worth starting somewhere as long as the product achieves a minimum level of usefulness.
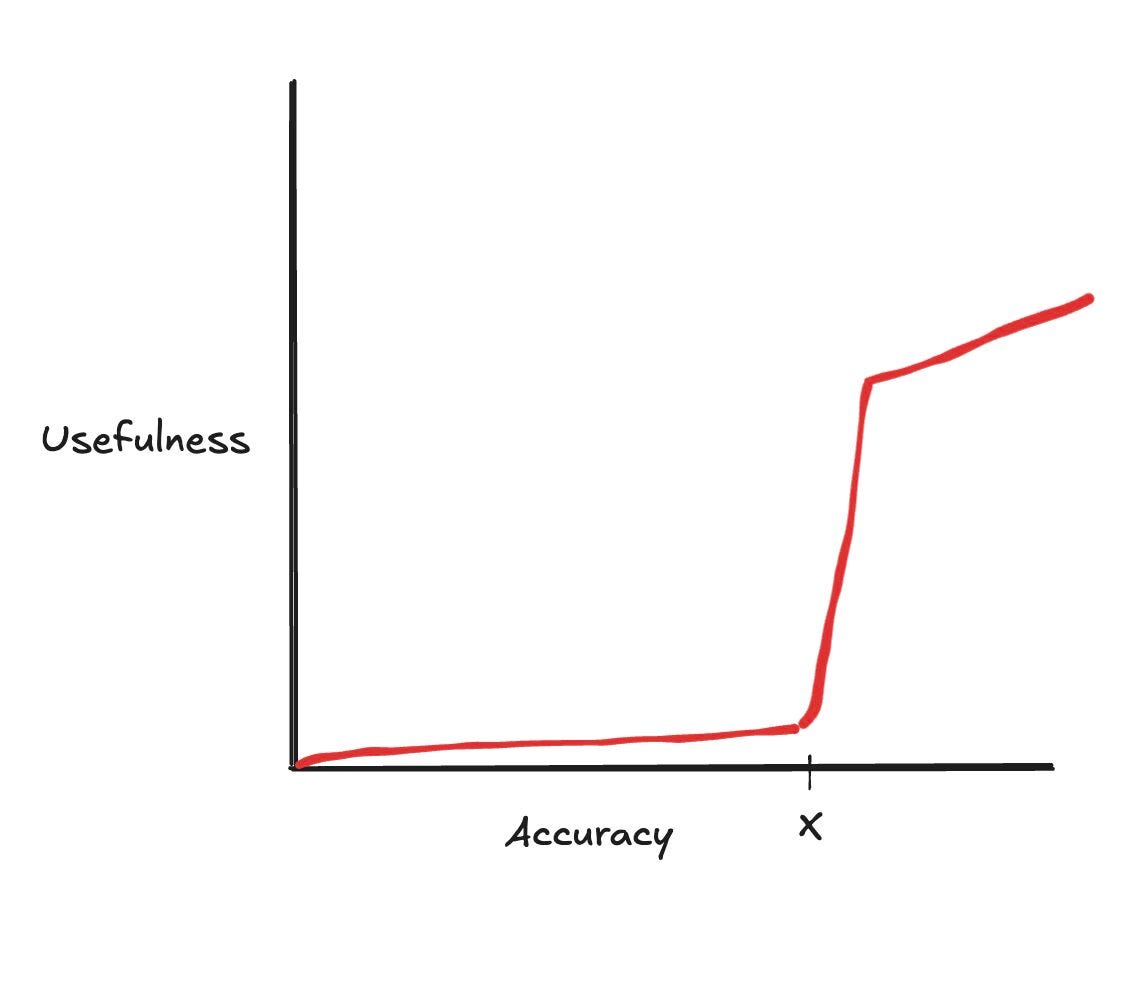
Even if you know the product you can deliver today is worse than what you could deliver in 2/5/10 years, you may only earn the right to sell that future product by starting now.
So, you should build the AI Sales Development Rep (SDR) or AI Recruiter, even if it is lackluster with GPT-4, as long as it meets a minimum usefulness threshold (like the 1988 Madden Game).
The leading AI SDR using GPT-4 (or equivalent) will be best positioned to win as GPT-5, GPT-6, and GPT-7 emerge. The talent, technology, and distribution around the initial product positions that company to capitalize on future technological leaps.
You can’t sit on the sidelines. The only way to win is to play the game.
To be clear: I’m still certainly far from an expert
“AI” is really an overly broad word. However, it’s unfortunately the word that we’ve collectively decided to use for anything from machine learning models of all kinds, computer vision, and Large Language Models. Here, I really mean the latest wave of Large Language Models.
In a fun coincidence, OpenAI’s latest fundraise values them just $5b short of AT&T’s current market cap ($150b vs $155b)
I say direct because NVIDIA has undoubtedly generated the most enterprise value from the transformer as a second order effect
https://www.independent.co.uk/news/science/apollo-11-moon-landing-mobile-phones-smartphone-iphone-a8988351.html
super insightful conclusions!